Predictive Analysis of Tourist Flows: from Data to Strategies
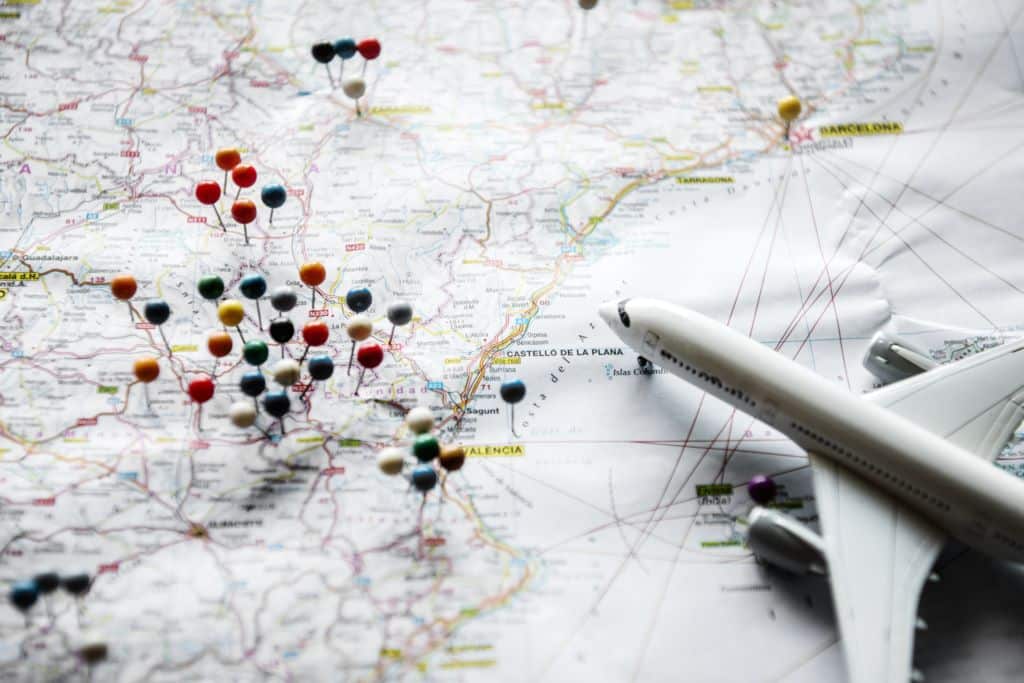
Predictive analysis is revolutionizing how we plan and manage tourism.
It’s no longer just about gathering historical data: today, thanks to Artificial Intelligence and advanced techniques, we can forecast and influence future tourist flows.
This ability to anticipate the needs and behaviours of visitors offers a significant competitive advantage for tourist destinations and industry operators.
In this post, we will explore how predictive analysis is being concretely applied in the tourism sector, with examples of real cases and innovative methodologies that are reshaping the landscape of modern tourism.
Geolocation Data for Predictive Analytics
One of the main challenges in predictive tourism analysis is the availability of accurate and comprehensive data.
However, a recent study has shown that reliable forecasts can still be obtained even with limited geolocation data, opening up new possibilities for destinations of all sizes.
In the past, less structured destinations didn’t have access to sufficient resources to collect and analyze complex data, but today, thanks to deep learning techniques, even small destinations can benefit from sophisticated predictive analysis.
The study “Prediction of Tourism Flow with Sparse Geolocation Data” (Lemmel et al., 2023), available at arxiv.org/abs/2308.14516, compared the effectiveness of different analytical approaches, demonstrating that deep learning models can be more effective than traditional statistical methods, even when data is sparse.
This is particularly significant for:
- Emerging destinations that are just beginning to collect data and do not yet have comprehensive databases.
- Areas with limited infrastructure for visitor monitoring, where data collection technology might be uneven or fragmented.
- Situations where data privacy is a major concern and the amount of data collected must be minimized.
Thanks to these models, it is possible to:
- Identify non-obvious tourist movement patterns, helping to better understand visitor behavior.
- Predict attendance peaks with greater precision, optimizing resource management.
- Optimize resources based on more accurate forecasts, ensuring that services and facilities are always ready to meet tourist demand.
Predictive Analysis of Flows Between Destinations
One of the most interesting developments in predictive analysis is understanding tourist flows between different destinations.
Often, tourists do not limit their visits to a single location but shift their interest to multiple destinations during the same trip. Understanding these movements allows for better planning and resource efficiency.
An innovative study, “Forecasting Inter-Destination Tourism Flow via a Hybrid Deep Learning Model” (Fang et al., 2023), available at arxiv.org/abs/2305.03267, has developed a hybrid model that analyzes tourists’ travel notes to predict movements between different destinations.
This model considers both the explicit characteristics of tourist attractions, such as their popularity or uniqueness, and the implicit interactions between the destinations themselves.
This approach is particularly effective in analyzing:
- Common travel patterns between different destinations, which can be leveraged to create integrated tourism packages.
- Destination combinations that are particularly popular among visitors, aiding in the development of strategic partnerships and collaborations between tourism entities.
- Average length of stay in each destination, allowing for tourism offerings to be better tailored to meet visitor expectations.
- Factors influencing itinerary choices, such as geographic proximity, transportation costs and availability, and related attractions.
Practical Applications of Predictive Analysis for Destination Managers
Thanks to this methodology, destination managers can:
- Develop strategic partnerships with other destinations to create more attractive offers and encourage tourists to extend their stays, visiting multiple locations.
- Create integrated itineraries that align with tourists’ preferred travel patterns, promoting a smoother flow management and increasing customer satisfaction.
- Optimize transportation services between destinations to enhance the overall visitor experience and reduce travel times, making the journey feel less stressful and more enjoyable.
- Customize tourism offerings based on predictable visitor movements, thereby improving the ability to provide relevant services and reducing the likelihood of service disruptions.
Social Media and Predictive Analysis
Social media represents an endless source of valuable data for understanding tourist preferences and intentions. Integrating social network analysis with semantic analysis is opening new frontiers in tourism demand forecasting.
A detailed study, “A social network and semantic analysis approach to predict tourism demand” (Fronzetti Colladon et al., 2019), available at arxiv.org/abs/2105.07727, has demonstrated how analyzing online travel forums can provide reliable predictive indicators for international tourist arrivals.
Innovative Methodology in Forecast Analytics
This study combines several analytical elements:
- The complexity of language used in forums is analyzed to identify emerging trends and better understand what draws tourists’ interest. Complex or highly descriptive language can indicate strong engagement and high interest in a destination.
- The centralization of the communication network helps in better understanding the influence of various actors in tourists’ decision-making processes and the spread of information. This aids in identifying influencers and key discussion points that drive destination choice.
Practical Outcomes of Predictive Analysis
This methodology has shown considerable predictive power for:
- International airport arrivals, helping destinations better prepare for peak periods and allocate resources more efficiently.
- Booking trends and interest levels in various destinations, providing useful insights for planning more effective promotional campaigns.
- Destination preferences and decisive factors in travel choices, such as the cost of stay, the variety of available activities, and the destination’s reputation.
Practical Guide to Predictive Analysis for Destination Managers
To successfully implement predictive analysis in a destination, it is essential to follow a structured yet flexible approach. Here are some key phases to maximize the potential of predictive analysis:
1. Preparation and Data Collection
- Evaluate available data sources: Such as geolocation data, social media and travel forums, historical visitor statistics, and booking data. Each data source contributes uniquely and helps build a more comprehensive view of visitor behavior.
- Integrate different data sources: It is important to combine data from various sources to achieve a more detailed and accurate picture of visitor behavior.
2. Selection of Predictive Analysis Tools and Methodologies
- For destinations with limited data: Use deep learning models optimized for sparse data, integrating alternative sources like social media. This allows for an effective starting point even with a less substantial data base.
- For more structured destinations: Develop hybrid models that combine various data sources and implement real-time analysis systems to respond quickly to changes in tourism demand.
3. Monitoring and Optimization
- Monitor forecast performance: Constantly compare forecasts with actual data to calibrate models. Continuous analysis allows for improved forecast accuracy and adaptation of operational strategies.
- Evaluate the impact on operational decisions: The true value of predictive analysis lies in its ability to guide resource allocation and enhance strategic decision-making. Monitoring how predictions concretely influence resource management is essential to demonstrate the value of this approach.
Implementing Predictive Analysis in Tourism
1. Gradual Approach to Predictive Analysis
- Pilot Phase: Start with a limited dataset and test models on a small scale, thoroughly documenting successes and challenges. This reduces risks and allows for learning from initial experiences before expanding implementation.
- Gradual Scaling: Progressively expand the scope of predictions, integrating new data sources and adjusting models based on stakeholder feedback, ensuring each phase adds value.
2. Training and Involvement of Stakeholders
- Staff Training: It is essential to train staff on the principles of predictive analysis, the use of visualization tools, and the interpretation of results. Only with adequate training can data be used to its full potential.
- Stakeholder Involvement: Regularly share results with local operators, gather feedback, and adjust predictions to meet operational needs to foster a data-oriented culture. This involvement helps build consensus and motivates all stakeholders.
The Future of Predictive Analysis in Tourism Flow Management
The evolution of predictive analysis in tourism is opening new opportunities to make destinations more competitive and sustainable:
- Integration with Artificial Intelligence: Increasingly sophisticated models allow for automated operational decisions and real-time personalization of tourism offerings, making visitor interactions smoother and more engaging.
- Real-time Analytics: Real-time forecasting enables immediate adaptation to market changes, continuously optimizing results and improving the ability to respond to tourists’ needs.
- Sustainability and Flow Management: Predictive analysis can contribute to a more sustainable management of tourist flows, avoiding overcrowding and distributing visitors more evenly across the territory, enhancing the quality of the experience and reducing environmental impact.
Conclusions and Recommendations on Predictive Analysis in Tourism
Predictive analysis is transforming how we manage tourist flows, providing destination managers with the ability to make more informed decisions and enhance visitor experiences. To maximize its benefits, it is essential to:
- Adopt a Strategic Approach, with clear objectives and a realistic roadmap guiding each phase of implementation.
- Focus on Continuous Innovation, experimenting with new models and integrating innovative data sources to constantly improve predictive capabilities.
- Value the Human Factor, combining predictive analysis with human experience and judgment to ensure high-quality and highly personalized service.
Predictive analysis is not merely a technological tool but a new way of thinking and managing tourism, capable of enhancing not only operational performance but also the quality of experiences offered to visitors. Investing in this technology means adopting a proactive approach, where every decision is data-driven and aimed at creating lasting value for all stakeholders involved.
***
Imagine: trip planning with map by Rawpixel
***
Bibliography
- (Fang et al., 2023) Fang, H., Gao, S., & Zhang, F. (2023). Forecasting Inter-Destination Tourism Flow via a Hybrid Deep Learning Model. arXiv:2305.03267 [cs.CY]. https://doi.org/10.48550/arXiv.2305.03267
- (Fronzetti Colladon et al., 2019) Fronzetti Colladon, A., Guardabascio, B., & Innarella, R. (2019). A social network and semantic analysis approach to predict tourism demand. Decision Support Systems, 123, 113075. https://doi.org/10.1016/j.dss.2019.113075
- (Lemmel et al., 2023) Lemmel, J., Babaiee, Z., Kleinlehner, M., Majic, I., Neubauer, P., Scholz, J., Grosu, R., & Neubauer, S. A. (2023). Prediction of Tourism Flow with Sparse Geolocation Data. arXiv:2308.14516 [cs.LG]. https://doi.org/10.48550/arXiv.2308.14516
***
Continue Exploring Innovation and Technology in Tourism!
If you want to dive deeper into innovation in tourism, I invite you to read my book: Andrea Rossi, “Digital Media and Tourism” (English Edition), 2024.
Available on Amazon worldwide!
Get your copy at:
- US -Amazon.com: https://www.amazon.com/dp/B0D1P49JNB/
- UK – Amazon.co.uk: https://www.amazon.co.uk/dp/B0D1P49JNB/
- IT – Amazon.it: https://www.amazon.it/dp/B0D1P49JNB/
- DE – Amazon.de: https://www.amazon.de/dp/B0D1P49JNB/
- ES – Amazon.es: https://www.amazon.es/dp/B0D1P49JNB/
- FR – Amazon.fr: https://www.amazon.fr/dp/B0D1P49JNB/
- NL – Amazon.nl: https://www.amazon.nl/dp/B0D1P49JNB/
Leave a Reply
Want to join the discussion?Feel free to contribute!